學術資源整合系統-相關推薦
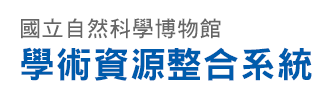 |
|
|
|
作者 | Wang;Pin-Wei |
出版日期 | 2022 |
已接受 | 202210 |
著作名稱 | SDSS-IV MaNGA: Unveiling Galaxy Interaction by Merger Stages with Machine Learning
|
刊名 | The Astronomical Journal |
卷 | 937 |
期 | 97 |
被收錄索引 | SCI;SCI |
主題 | 天文;天文 |
摘要 | We use machine-learning techniques to classify galaxy merger stages, which can unveil physical processes that drive the star formation and active galactic nucleus (AGN) activities during galaxy interaction. The sample contains 4690 galaxies from the integral field spectroscopy survey SDSS-IV MaNGA and can be separated into 1060 merging galaxies and 3630 nonmerging or unclassified galaxies. For the merger sample, there are 468, 125, 293, and 174 galaxies (1) in the incoming pair phase, (2) in the first pericentric passage phase, (3) approaching or just passing the apocenter, and (4) in the final coalescence phase or post-mergers. With the information of projected separation, line-of-sight velocity difference, Sloan Digital Sky Survey (SDSS) gri images, and MaNGA Hα velocity map, we are able to classify the mergers and their stages with good precision, which is the most important score to identify interacting galaxies. For the two-phase classification (binary; nonmerger and merger), the performance can be high (precision > 0.90) with LGBMClassifier. We find that sample size can be increased by rotation, so the five-phase classification (nonmerger, and merger stages 1, 2, 3, and 4) can also be good (precision > 0.85). The most important features come from SDSS gri images. The contribution from the MaNGA Hα velocity map, projected separation, and line-of-sight velocity difference can further improve the performance by 0%–20%. In other words, the image and the velocity information are sufficient to capture important features of galaxy interactions, and our results can apply to all the MaNGA data, as well as future all-sky surveys. |
系統號 | NO000006561 |
|
|